Cognitive Appeals Automation Cuts Costs by 50%, Spikes Star Ratings for Major Payer
A leading national health insurance company used Sagility’s intelligent machine learning solution, leading to cognitive appeals automation cutting costs by up to 50%.
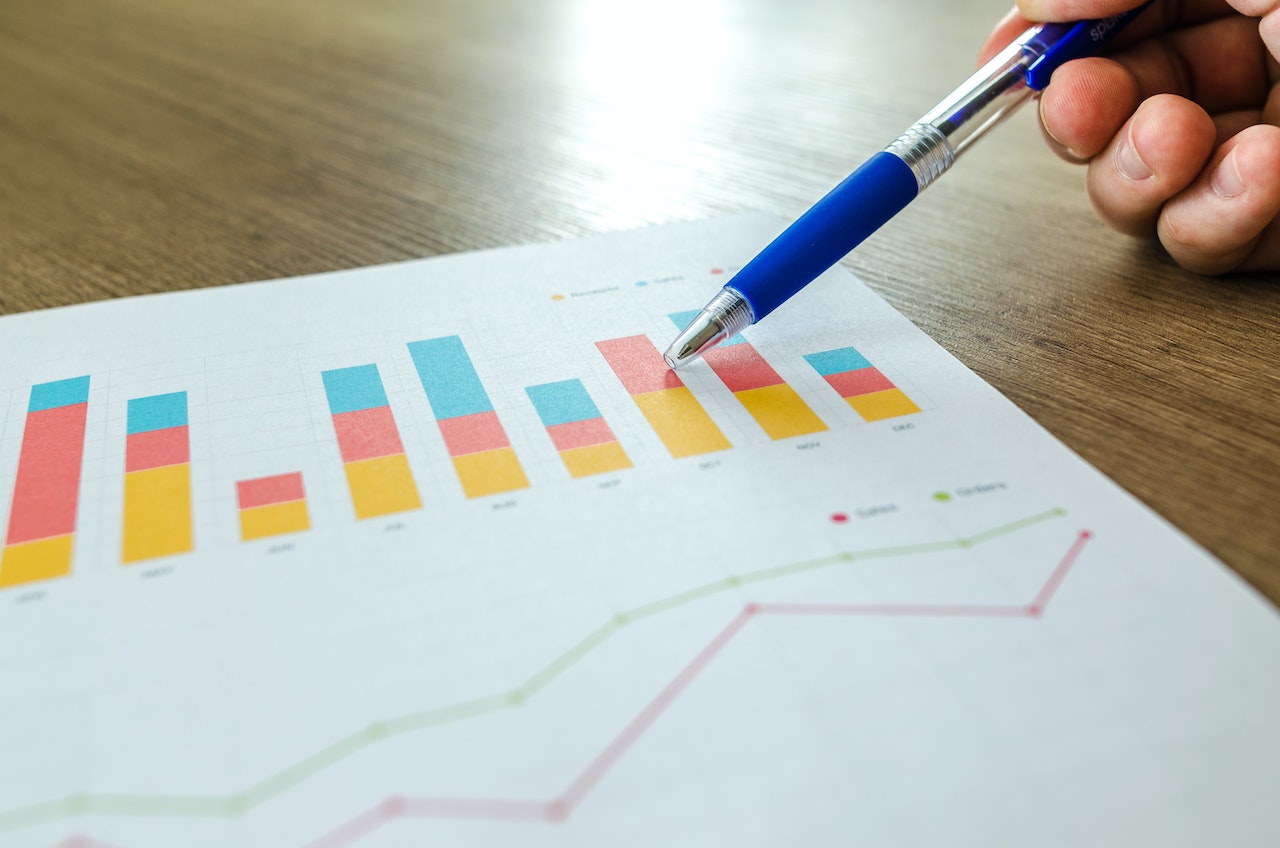
About the Client
The Fortune 500 client is one of the largest health insurance companies in the U.S.
Balancing Costs with Customer Experience
The client had several disparate, manual applications relying on a human agent to pull data to deliver a service intake of faxes and scanned document images into manual queues. The inputs consisted of different sources and format types, and working through repetitive processes was a less-than-optimal use of employees’ time. As a result, the client was facing an 84% upheld rate of denial decisions in several markets. With the client’s average auto-forward rate of four per month, this led to a negative impact on their Star ratings. There were over 5,000 claims per week, and seasonality in data added further complexities to the issue.
Improved Intake Process with AI Automations
Sagility delivered an intelligent machine learning solution to help improve the client’s Star rating, specifically by improving grievances and appeals efficiencies. The team addressed the entire workflow with a cognitive content processing solution, leveraging an image analytics engine to process the source. The solution featured a natural language processing (NLP) engine to analyze keywords and context in client grievances and appeals correspondence.
Documents were classified as expedited or non-expedited on the basis of rules or associate judgment. The solution leveraged an NLP engine to minimize subjectivity and improve process efficiency and addressed all types of text—unstructured, semi-structured, and fully structured. The NLP engine sent the documents to the machine learning text classifier, which tagged and queued documents for further processing. With self-learning, this cognitive solution recognized patterns of subjectivity and replicated human-associated decision-making over time.
The process re-engineering solution included the following:
• Intelligent content processing through developed internal image analytics engine for source and format-agnostic
extraction and processing and processing of unstructured, semi-structured, or fully structured documents
• NLP engine to analyze keywords, context, and intent in the documents
• ML engine to classify the documents based on image analytics and NLP to push the documents into the required queue
RESULTS
The project was launched within two months with an objective of reducing overturned denial decisions and
auto-forward rates by 50–75%. While the solution has enabled significant process enhancements, it also
offers additional value from all the case data gathered. The system analyzes exceptions in cases from the
past to determine the direction of the workflow through a feedback learning loop. The loop helps tackle similar scenarios in the future, which ensures that case exceptions are always taken care of without requiring human intervention. Key outcomes of the Sagility solution included:
4 Star
Measure Areas Improved
Namely Appeals Auto- Forward, Appeals Upheld, Timely Decisions about Appeals, and Reviewing Appeals Decisions
30-50%
Reduction
In FTEs
0
Average Auto-Forwards
Which improved the Star ratings of the MA plan by an 8% weighted average
40-50%
Improvement
In workflow efficiency
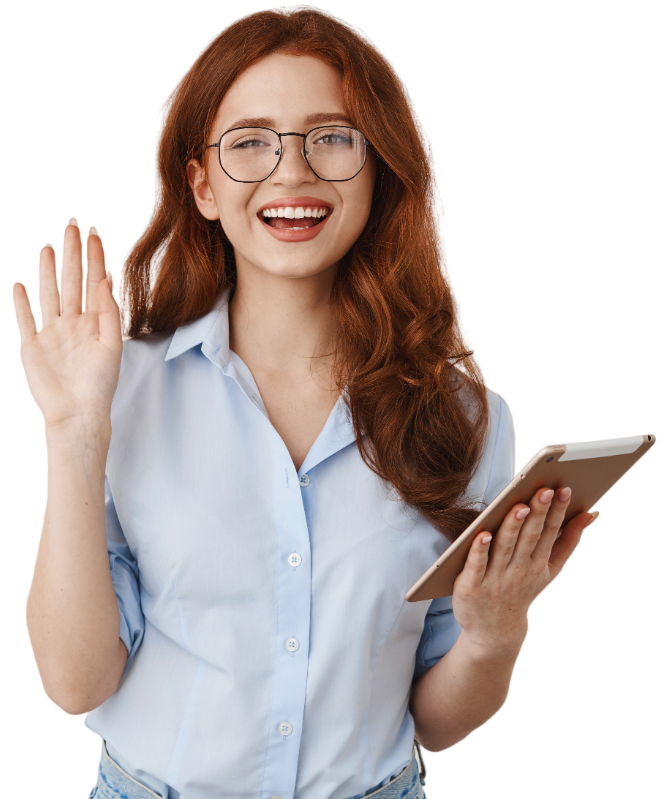